Key Resources
Looking for more information? Here’s everything you should need.
Press Release
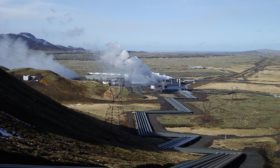
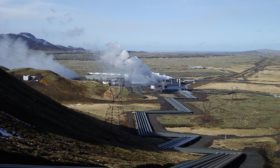
40% of China’s coal power stations are losing money
Carbon Tracker launches revolutionary satellite-based methodology to assess climate risk from fossil fuel...
Read MoreIn this report, we present the results from a revolutionary method pioneered by Carbon Tracker to use satellite imagery to estimate the utilisation of fossil fuel power plants.
Using advanced machine learning techniques to process both commercial and public satellite images of coal plants, we explore the potential to estimate the capacity factors of coal plants in regions where data is inadequate for investors, policymakers and campaigners to make informed decisions. This analysis brings asset-level data to life by making it possible to develop actionable climate scenario analysis for coal power. It can also help investors gain trading insights in emerging Asian economies, which are significant contributors to global GDP and have considerable fossil fuel exposure.
Key Findings
Space revolution – data provision is going to improve in ways previously unimaginable
There is a revolution happening in space. As of August 2017, there were nearly 1,800 operational satellites in orbit. Of these, 596 are used for earth observation. Planet, a commercial satellite provider, has over 175 satellites in orbit, enabling them to produce an image anywhere on the globe with up to 30 cm resolution. The space revolution is part of a wider digital revolution coursing through every sector of the economy from manufacturing to finance – cloud computing, predicative analytics and remote sensing – and transforming the power sector as well. This paradigm shift in the availability, coverage and granularity of satellite imagery coupled with advances in machine learning are going to have profound implications for asset-level data and environmental monitoring.
Asset utilisation – a vital and elusive variable
Plant utilisation is essential for economic analysis of power generation assets, as plants derive the majority of their revenues through the volume of electricity they produce and sell through tariffs and wholesale pricing. However, in many power markets outside the EU and the US, plant utilisation data is often untimely, unreliable or publicly-unavailable. These markets, where governments and asset owners are either unable or unwilling to provide granular and timely data, are predicted to dominate global demand growth for both power generation and fossil capacity. For instance, according to the International Energy Agency (IEA), China could represent around one-third of power generation and over 20% of fossil fuel-based capacity globally by 2040. The Paris Agreement requires a relatively rapid phaseout of coal fleet by 2040. Decision-makers need support to ensure an orderly and economically rational phase-out. Asset-level data and modelling lies at the heart of these retirement schedules. Our methodology can improve the data provision and accuracy of asset-level modelling.
Nowhere to hide – model accuracy of ~90%
Our modelling techniques showed that satellite imagery can independently derive coal power plant capacity factors when aggregated at local levels. For instance, the average precision of our model for predicting capacity factors of US and EU plants was 91% and 92%, respectively. Modelling accuracy was strongly influenced by a number of factors, including:
(i) available images due to both cloud cover and satellite revisit rate;
(ii) differences in cooling technology between plants; and
(iii) the size of the plant.
Nonetheless, these results suggest that a quantitative methodology to estimate the capacity factor using satellite imagery is potentially feasible. As the quality of satellite imagery improves, we expect our methodology to be a powerful tool for investors, policymakers and campaigners to navigate the transition away from fossil fuel power generation.
Making asset-level data actionable – shining a spotlight on China
As decision-makers turn their focus towards phasing-out existing fossil fuel power capacity, the data requirement will need to become timelier and more accurate. For example, the IEA, stated in 2015 that Chinese coal demand would peak in 2030 – only to say in 2016 that Chinese coal demand had already peaked in 2013. Moreover, for asset-level inventory data to be actionable it will need to be translated into economic and financial outcomes. We applied our satellite imagery methodology and integrated the plant utilisation results at the local level to our ‘investment grade’ climate scenario analysis for coal power in China, of which provides insight into when coal plants should close and the potential stranded asset risk of delaying their retirement.
The headline findings from our coal power analysis for China, include:
- Owing to high fuel costs (capacity-weighted average of $85/t), 40% of the coal fleet may be cash-flow negative in 2018 and due to carbon pricing ($40/t by 2040) and air pollution regulation 95% of the fleet could be cash-flow negative by 2040;
- It will be cheaper to build new onshore wind than operate coal by 2021 and solar PV by 2025; and
- As the majority of units are loss-making from 2018 to 2040, Chinese coal power owners can avoid losing $390 bn by retiring the operating fleet in a manner consistent with the Paris Agreement. Cost-optimised retirement schedules show Guangdong Yudean Group, Zhejiang Energy Group and National Energy Investment Group could benefit the most from premature closures consistent with Paris, with asset stranding as a percentage of total capital of 117%, 101% and 52%, respectively.